The afternoon I meet Dexter Hadley, the haze from Camp Fire, 160 miles to the northeast, is thick enough to dim the afternoon sun. Hadley is a physician and scientist at the University of California, San Francisco, and for lunch we go to a food-truck gathering spot near his office. Under the circumstances, the small gas-burning fire pit in the center of the space seems superfluous, but its Mad Max ambiance—and the many people walking the streets wearing surgical masks—lends a suitably apocalyptic air to our discussion. It’s a good day to talk about tearing down the entrenched structures of public health.
Hadley has the smooth voice of a late-night DJ. He likes to say that ten years of medical school helped him figure out how not to practice medicine. “I went to a top-three medical school and I didn’t hear the word ‘genome’ once,” he says (even though genomic medicine is a hot topic these days). After earning an MD, and then a PhD in genomics and computational biology (with a Masters in systems engineering thrown in for good measure), Hadley took a residency in pathology at Stanford. In his downtime, he took a class in startup engineering that asked students to devise crowdfunding plans using bitcoin. “Clearly,” he says, “I was trying not to do pathology.”
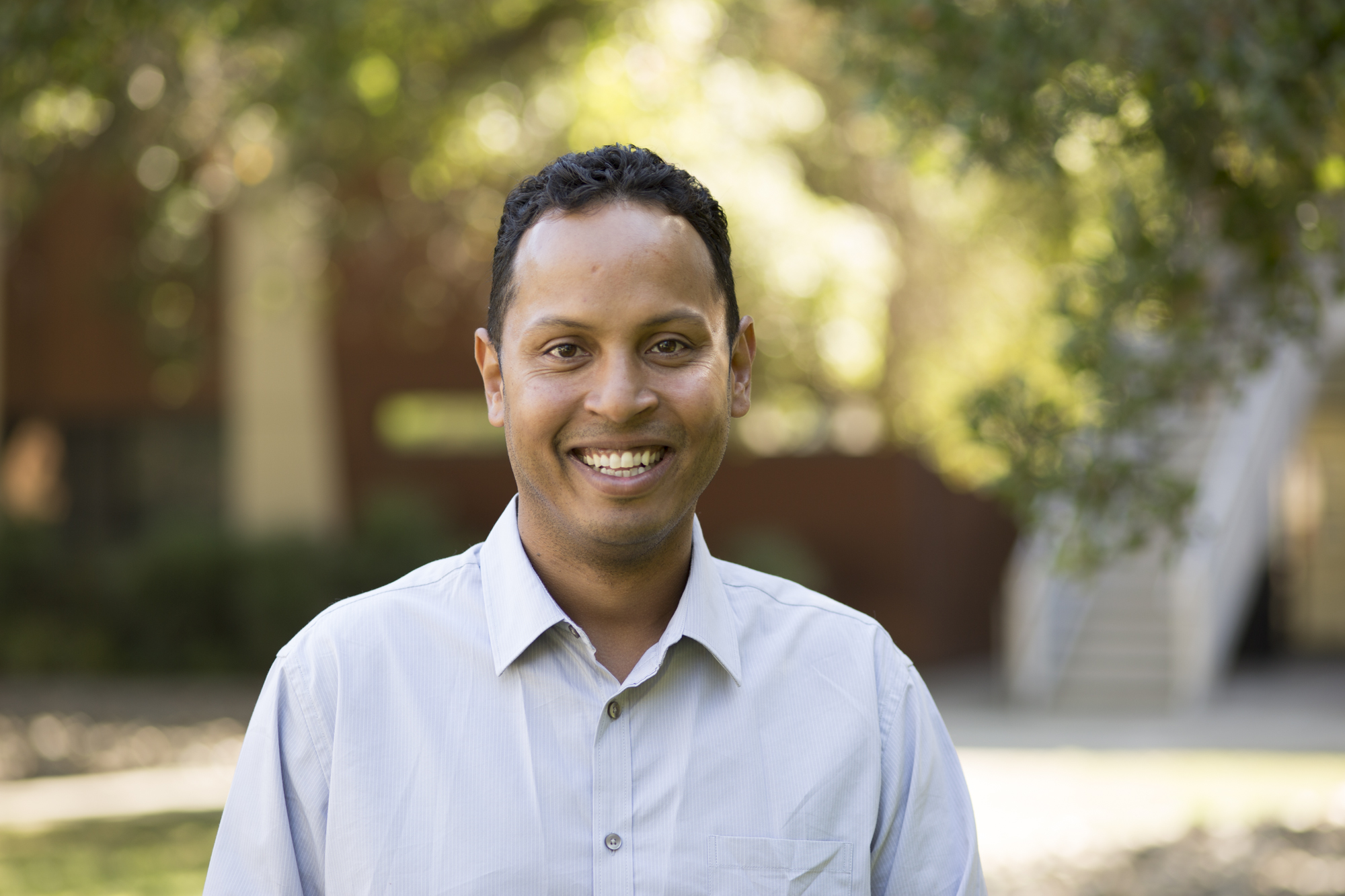
Now 40, Hadley runs a lab at UCSF that studies how big data can improve medical studies. Finally, he has found something he does want to do, which is to use deep learning and blockchain to conquer a horrible disease. His plan is to amass enough mammograms, so he can train artificial intelligence algorithms to detect breast cancer (his site BreastWeCan is already crowdsourcing clinical images). The blockchain aspect is to secure health data, so it belongs to individuals who can profit from that data, rather than the large health institutions that currently control it.
The world of medicine is starting to take deep learning seriously because it’s started to become useful. Until recently, computers were no good at making the visual distinctions that we take for granted—like seeing a cat and knowing it’s not a dog. But now A.I. platforms can “see” better than us. “Today, if you have a dark, low-resolution image of a cat,” Hadley told an audience in September at the Heidelberg Laureate Forum in Germany, “Google has a better chance of knowing it’s a cat than a human.” This visual acuity enables computers to beat doctors in detecting skin cancer lesions and some ophthalmologic conditions, he says.
Breast cancer makes a natural test-case for Hadley’s lab, because it is a disease that is both common and widely misdiagnosed. One out of every eight women will be diagnosed with breast cancer, but almost all women—95 percent—will at some point be given a false diagnosis. The mammogram—the universally accepted method of detecting breast cancer—is barely better at finding cancer than self-examination. “Mammography is a ten-billion-dollar industry devoted to something that doesn’t work,” Hadley says. “That’s not my opinion—that’s reality. It just doesn’t have the public health value we expect.”
“Health data is the most valuable data in the world, and the patient gets no return on it, except for the treatments.”
The information is often there on the mammograms. But even the most experienced doctors do not have the native ability to interpret it. Hadley believes that deep learning programs, which get more accurate and powerful the more examples of something they come across, can learn to see cancer when it is there, and not be fooled, like a mammogram, when it is not. He just needs a sufficiently large trove of data to test his thesis—five million mammograms should do it perhaps.
Therein, though, lies the problem. Health data is hoarded by large institutions that collect it. In much the same way that Google and Facebook don’t like to share data with their competitors, major players in health care, like hospital groups and pharmaceutical companies, are fond of stockpiling patient data and not making it available for research. In part, this behavior is mandated by federal privacy laws, like the Health Insurance Portability and Accountability Act (HIPAA). But Hadley says privacy is mostly an excuse; the real motivation is making money.
Putting the world’s mammograms on an open blockchain would not only provide a rich data source for researchers. It might also reward patients for what is really theirs. And since individuals would control their own data, those who want to use it would presumably offer to buy it. The stockpiling and siloing of this data already demonstrates its value.
“Health data is the most valuable data in the world, and the patient gets no return on it, except for the treatments,” Hadley says.
If it works, Hadley thinks his incentive-based system will result in a data-set reflective of the wider patient base in the U.S. A marketplace will encourage everyone to come forward with their health information, and hopefully bring to the fore the rarer cancer cases most valuable to researchers. Ideally, the collection will include mammograms that doctors interpreted correctly, yielding correct positive or negative diagnoses, but also those that yielded false positives or negatives.
Hadley wants to revolutionize the medical industry’s treatment of medical data as much as its treatment of diseases. He’s certainly not the only doctor using A.I. to fight breast cancer, or the first to propose using blockchain to make give patients more control of their medical data. But he may be the first to unite the two concepts.
“We have a country with millions of people, but data is treated like illicit drugs,” he says. “If you want to buy a kilo of cocaine, you do it behind closed doors. It’s the same if you want to buy data from Sloan-Kettering. The patient benefits nada at the moment.”